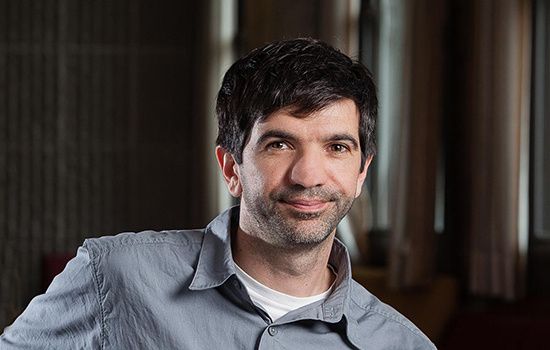
DDLC Seminar: Serdar Yüksel (Queen’s)
Watch on YouTube Live
Robustness to Approximations, Learning, and Incorrect Models in Stochastic Control
In stochastic control, typically, an ideal model is assumed or an estimate model is learned, and the control design is based on this model, raising the robustness problem of performance loss due to the mismatch between the assumed model and the actual system. Even when a correct model is available, computation constraints may dictate the use of approximation methods. In this talk, we will view approximations and robustness under a unified theme. Robustness may be with regard to the system model, driving noise distribution, the initial prior, or approximations as required by computational methods. We will study this problem in both discrete-time and continuous-time and under several criteria and information structures.
Bio:
Serdar Yüksel received the B.Sc. degree in electrical and electronics engineering from Bilkent University, Ankara, Turkey, in 2001 and the M.S. and Ph.D. degrees in electrical and computer engineering from the University of Illinois at Urbana-Champaign, Champaign in 2003 and 2006, respectively. He was a Postdoctoral Researcher at Yale University before joining Queen’s University, Kingston, ON, Canada, as an assistant professor of mathematics and engineering in the Department of Mathematics and Statistics, where he is currently an associate professor. He has held visiting positions at KTH Royal Institute of Technology, Stockholm, Sweden and Bilkent University. He received the 2013 CAIMS/PIMS Early Career Award in applied mathematics and is currently an associate editor for the IEEE Transactions on Automatic Control. His research interests include stochastic control, information theory, probability, and networked control.