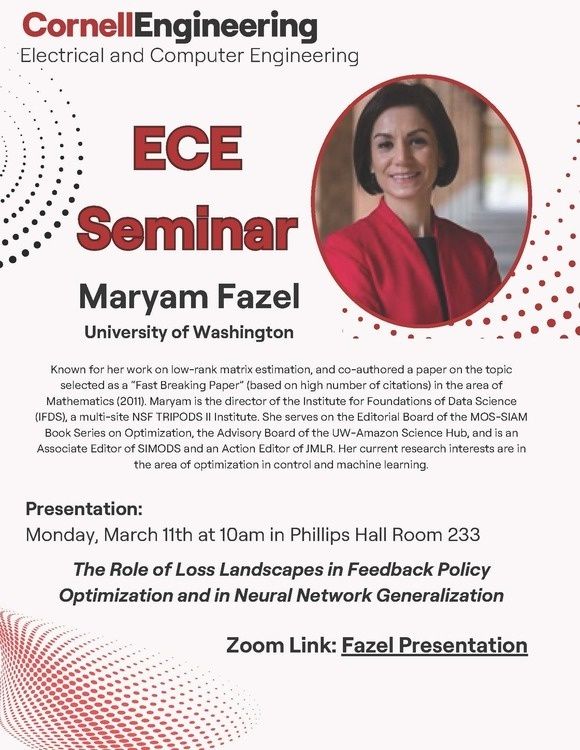
ECE Seminar: Maryam Fazel, University of Washington
Title: The Role of Loss Landscapes in Feedback Policy Optimization and in Neural Network Generalization
Abstract: The first part of this talk focuses on recent developments in policy optimization (a gradient-based iterative approach for feedback control design) popularized by the success of reinforcement learning (RL). We prove the global convergence of policy optimization in several canonical continuous control problems, despite their nonconvexity in policy parameters, by exploiting structural properties such as the PL condition. This line of work attempts to bring control theory and RL closer, and has led to two recent trends: (1) use of control problems as benchmarks for less-understood RL algorithms, and (2) theoretically-sound use of RL-style methods in control.
In the second part, we shift attention to a core question in deep learning: when does an overparameterized neural network generalize? Empirical evidence suggests generalization depends on which minimum is attained during training: “flat” minima appear to generalize well. We focus on a class of nonconvex models arising in low-rank matrix recovery as simple test cases, and prove that for several well-known models under standard statistical assumptions (e.g., matrix sensing, matrix completion, robust PCA, and two-layer neural networks), flat minima (those with smallest local average curvature) do generalize. This algorithm-agnostic result suggests a theoretical basis for favoring methods that bias iterates towards flat solutions.
Bio: Maryam Fazel is the Moorthy Family Professor of Electrical and Computer Engineering at the University of Washington, with adjunct appointments in Computer Science and Engineering, Mathematics, and Statistics. Maryam received her MS and PhD from Stanford University, her BS degree from Sharif University of Technology in Iran, and was a postdoctoral scholar at Caltech before joining UW. She is a recipient of the NSF Career Award, UWEE Outstanding Teaching Award, a UAI conference Best Student Paper Award with her student. She is known for her work on low-rank matrix estimation, and co-authored a paper on the topic selected as a “Fast Breaking Paper” (based on high number of citations) in the area of Mathematics (2011). Maryam is the director of the Institute for Foundations of Data Science (IFDS), a multi-site NSF TRIPODS II Institute. She serves on the Editorial Board of the MOS-SIAM Book Series on Optimization, the Advisory Board of the UW-Amazon Science Hub, and is an Associate Editor of SIMODS and an Action Editor of JMLR. Her current research interests are in the area of optimization in control and machine learning.