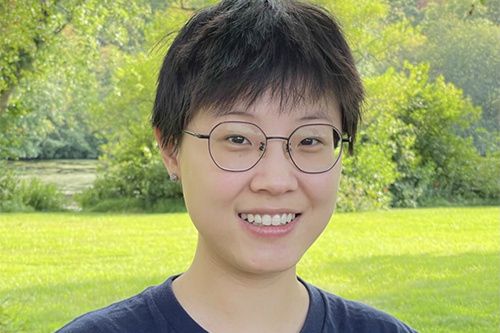
ORIE Colloquium: Shuangping Li (Stanford)
Spectral Clustering in the Gaussian Mixture Block Model
The Gaussian Mixture Block Model is a generative model for networks with community structure, designed to better capture structures observed in real-world networks. In this model, each vertex is associated with a latent feature vector, which is sampled from a mixture of Gaussians. These Gaussian components correspond to distinct communities within the network. Between each pair of vertices, an edge is added if and only if their feature vectors are sufficiently similar.
In this talk, I will present an efficient spectral algorithm for clustering (inferring community labels) and embedding (estimating latent vectors). My focus will be on the high-dimensional regime, where the latent feature space is high-dimensional – a setting that is both relevant to modern networks and mathematically challenging. For embedding, I will demonstrate that, provided the graph is not too sparse and the dimensionality is not excessively high, the spectral algorithm delivers accurate estimates of the latent vectors. Furthermore, for clustering, when the separation between communities is sufficiently large, the spectral algorithm enables the recovery of the communities. I will also discuss corresponding impossibility results, highlighting conditions under which these tasks become infeasible, thereby rendering our results sharp up to logarithmic factors.
Bio: Shuangping Li is a Stein Fellow in statistics at Stanford University. She earned her Ph.D. in applied and computational mathematics from Princeton University under the guidance of Professors Allan Sly and Emmanuel Abbe. Her research lies at the intersection of probability theory, theory of algorithms and complexity, high dimensional statistics, and theoretical machine learning.