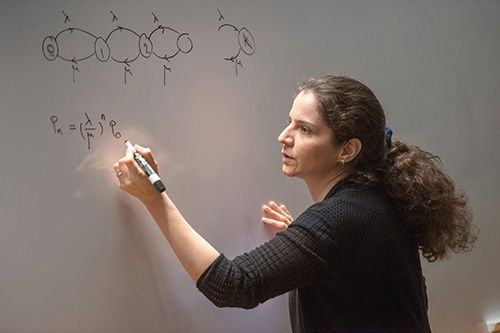
ORIE Colloquium: Vahideh Manshadi (Yale)
Dynamic Matching with Post-allocation Service and its Application to Refugee Resettlement
Motivated by our collaboration with a major refugee resettlement agency in the U.S., we study a dynamic matching problem where each new arrival (a refugee case) must be matched immediately and irrevocably to one of the static resources (a location with a fixed annual quota). In addition to consuming the static resource, each case requires post-allocation service from a server, such as a translator. Given the time-consuming nature of service, a server may not be available at a given time, thus we refer to it as a dynamic resource. Upon matching, the case will wait to avail service in a first-come-first-serve manner. Bursty matching to a location may result in undesirable congestion at its corresponding server. Consequently, the central planner (the agency) faces a dynamic matching problem with an objective that combines the matching reward (captured by pair-specific employment outcomes) with the cost for congestion for dynamic resources and over-allocation for the static ones. Motivated by the observed fluctuations in the composition of refugee pools across the years, we design algorithms that do not rely on distributional knowledge constructed based on past years’ data. To that end, we develop learning-based algorithms that are asymptotically optimal in certain regimes, easy to interpret, and computationally fast. Our design is based on learning the dual variables of the underlying optimization problem; however, the main challenge lies in the time-varying nature of the dual variables associated with dynamic resources. To overcome this challenge, our theoretical development brings together techniques from Lyapunov analysis, adversarial online learning, and stochastic optimization. On the application side, when tested on real data from our partner agency, our method outperforms existing ones making it a viable candidate for replacing the current practice upon experimentation.
Bio: Vahideh Manshadi is the Michael H. Jordan Professor of Operations at Yale School of Management and the research director for operations research at the Center for Algorithms, Data, & Market Design at Yale. Her current research focuses on the operation of online and matching platforms in both the private and public sectors. Her research has been recognized by numerous awards across various INFORMS communities, including Auctions & Market Design, Public Sector OR, Service Science, and Manufacturing & Service Operations Management. Manshadi serves on the editorial boards of Management Science and Operations Research and as the vice president of the Auctions & Market Design section at INFORMS. She received her Ph.D. in electrical engineering at Stanford University, where she also received M.S. degrees in statistics and electrical engineering. Before joining Yale, she was a postdoctoral scholar at the MIT Operations Research Center.