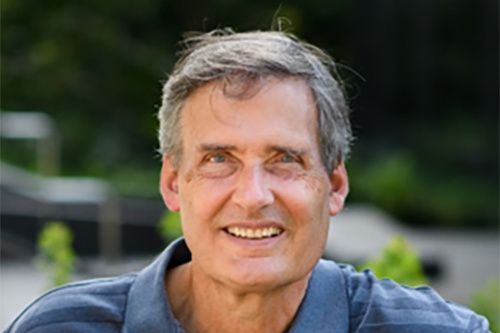
ORIE Colloquium: Warren Powell (Princeton)
A Fresh Approach to Teaching Optimization: From Deterministic Optimization to the Jungle of Stochastic Optimization
Operations research/industrial engineering departments have long taught deterministic optimization using a standard format, whereas decisions that involve uncertainty are taught in a wide range of styles that I have been calling the “jungle of stochastic optimization.” I am going to make the argument that most (almost all?) deterministic optimization problems are actually instances of fully sequential decision problems. I will present a universal framework for modeling all sequential decision problems, which means some form of stochastic optimization. I am then going to argue that this material can and should be taught broadly, including to undergraduates as well as graduate students outside of the analytically sophisticated fields.
The problems with teaching optimization are not limited to OR/IE departments. This spans optimal control (taught in other engineering departments), stochastic control (economics/finance), and reinforcement learning (computer science, psychology). Equally problematic are all the departments that do not even teach a formal optimization course (chemistry, biology, physics, politics, sociology…).
“Optimization” should be the science of making the best possible decisions, and not just problems that require sophisticated math and algorithms. Making decisions is a universal activity, and yet we teach it in a style that is limited to analytically sophisticated communities. Contrast this with how statistics (aka data sciences) is taught to graduates and undergraduates with a wide range of analytical skills.
A byproduct of our emphasis on mathematics is that we have failed to teach our students how to model complex problems. We think of a decision as “x” but just what is “x” if we are trying to minimize deaths from overdoses or disease, address climate change problems or create robust supply chains? I will make the case that we need to train students in problem domains how to frame problems in a form that sets the stage for mathematical models, whether or not they are needed.
Bio: Warren B. Powell is Professor Emeritus at Princeton University, where he taught for 39 years, and is currently a co-founder and Chief Innovation Officer at Optimal Dynamics as well as Executive-in-Residence at Rutgers Business School. He was the founder and director of CASTLE Lab, which focused on stochastic optimization with applications to freight transportation, energy systems, health, e-commerce, finance and the laboratory sciences, supported by over $50 million in funding from government and industry. He has pioneered a new universal framework that can be used to model any sequential decision problem, including the identification of four classes of policies that spans every possible method for making decisions. This is documented in his latest book with Wiley: Reinforcement Learning and Stochastic Optimization: A unified framework for sequential decisions. He published over 250 papers, five books, and produced over 60 graduate students and post-docs. He is the 2021 recipient of the Robert Herman Lifetime Achievement Award from the Society for Transportation Science and Logistics, the 2022 Saul Gass Expository Writing Award. He is a fellow of Informs, and the recipient of numerous other awards.